CNN(LeNet-5) & MNIST
The objective of this project is the same as seen in Multilayer Perceptron (MLP) & MNIST but with a difference in the use of operations called image convolutions. These networks are trained with images to extract features through convolutions in order to classify the numbers from the MNIST database.
Dataset MNIST

The public database MNIST [1] consists of 70,000 data, of which are already divided into 60,000 for training and 10,000 for testing. This data is a set of real numbers (0 to 9) handwritten by a human that is in a 28 x 28 grayscale format.
Data preparation
From the database, the training and test sets are downloaded to later be resized and normalized. Then the training set is divided into test and validation sets, in order to verify the behavior of the network. The separation of the data was done under the 60,20,20 rule.
Download data in training and test set
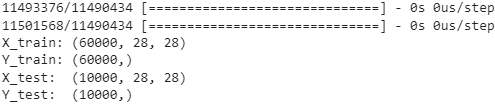
Resizing


Normalization
Splitting data based on Rule 60,20,20

Data preparation
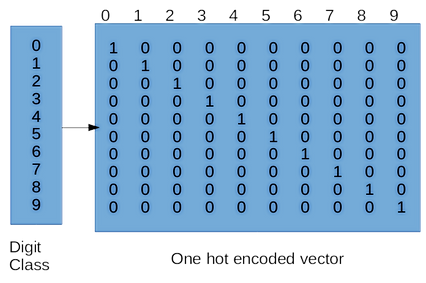
One-hot encoding
'one-hot' encoding is a tool that will allow us to value positions according to their numerical value. Each position of the coding being a neuron that will give a probability to that position or the numerical value; in such a way that of the 10 neurons (in this case) only one will have the highest probability and that will be the prediction.
Building of the LeNet-5 model
Se construyo el modelo de forma secuencial siguiendo la estructura de esta la primera red CNN.

Training the model
The training of the model counts on assigning certain hyperparameters that make the network learn in a better or worse way, this training was carried out by periods and only uses the training and validation sets. These two sets are used to compare them with each other and see the behavior of the network.

Results
Once the model has been trained, it is evaluated against the corresponding data sets, so the model is 0.97 or 97.0% accurate, which is an excellent value, clearly outperforming the MLP model. And in the figure below you can see an image taken randomly from the test set along with its prediction.


For more details, you can see the report here.
Annexes
Here you can see a classification of the status of a pedestrian (crossing or not crossing) using the same CNN-LeNet-5, demonstrating that it can be applied to many more similar or even different tasks.
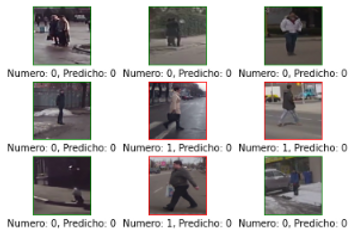